Getting bored of PowerPoint?
Experience the Prezi Difference
Experience the Prezi Difference
We help you stand out from the crowd and create a lasting impression, fast.
See how Prezi is better
Advanced AI features
Prezi AI brings your ideas to life in seconds. Unlike PowerPoint AI tools that offer a generic, one-size-fits all solution, Prezi AI lets you tailor your presentation to your liking. Select your background image, color palette, and design with the help of a true design assistant and watch your ideas come to life.
Want to learn more about Prezi AI?
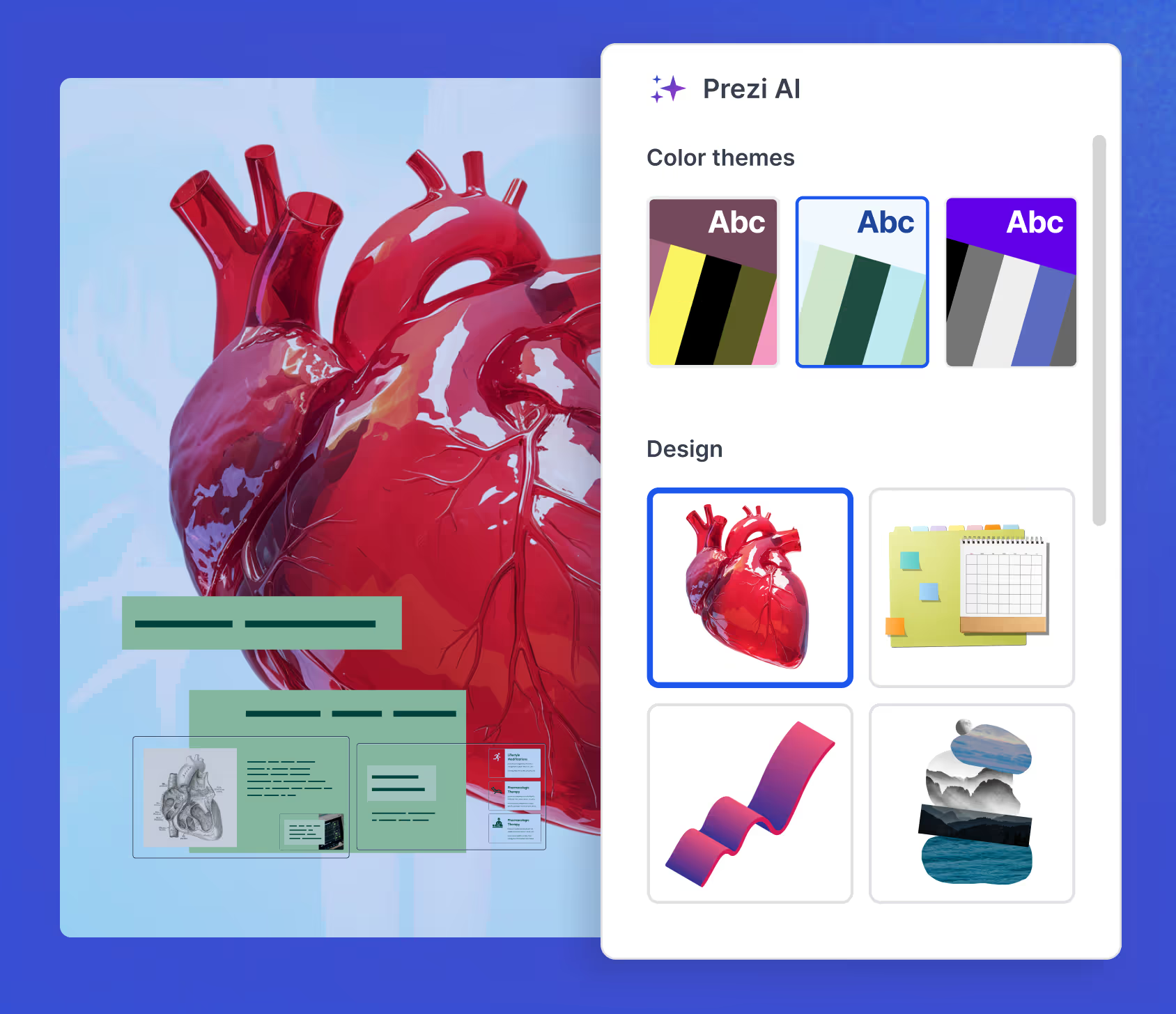
Proven increased engagement
A study comparing Prezi with traditional slide-based options like PowerPoint presentations revealed that Prezi is:
12.5%
more organized16.4%
more engaging21.9%
more persuasive25.3%
more effective overall
All those numbers add up to one thing:
better presentations with Prezi.
Designed for creative storytelling
Prezi helps you tell a cohesive story and create visual relationships that break away from the slide-by-slide bore. Go where your audience wants to with our open canvas that allows you to present conversationally and add objects anywhere you want.
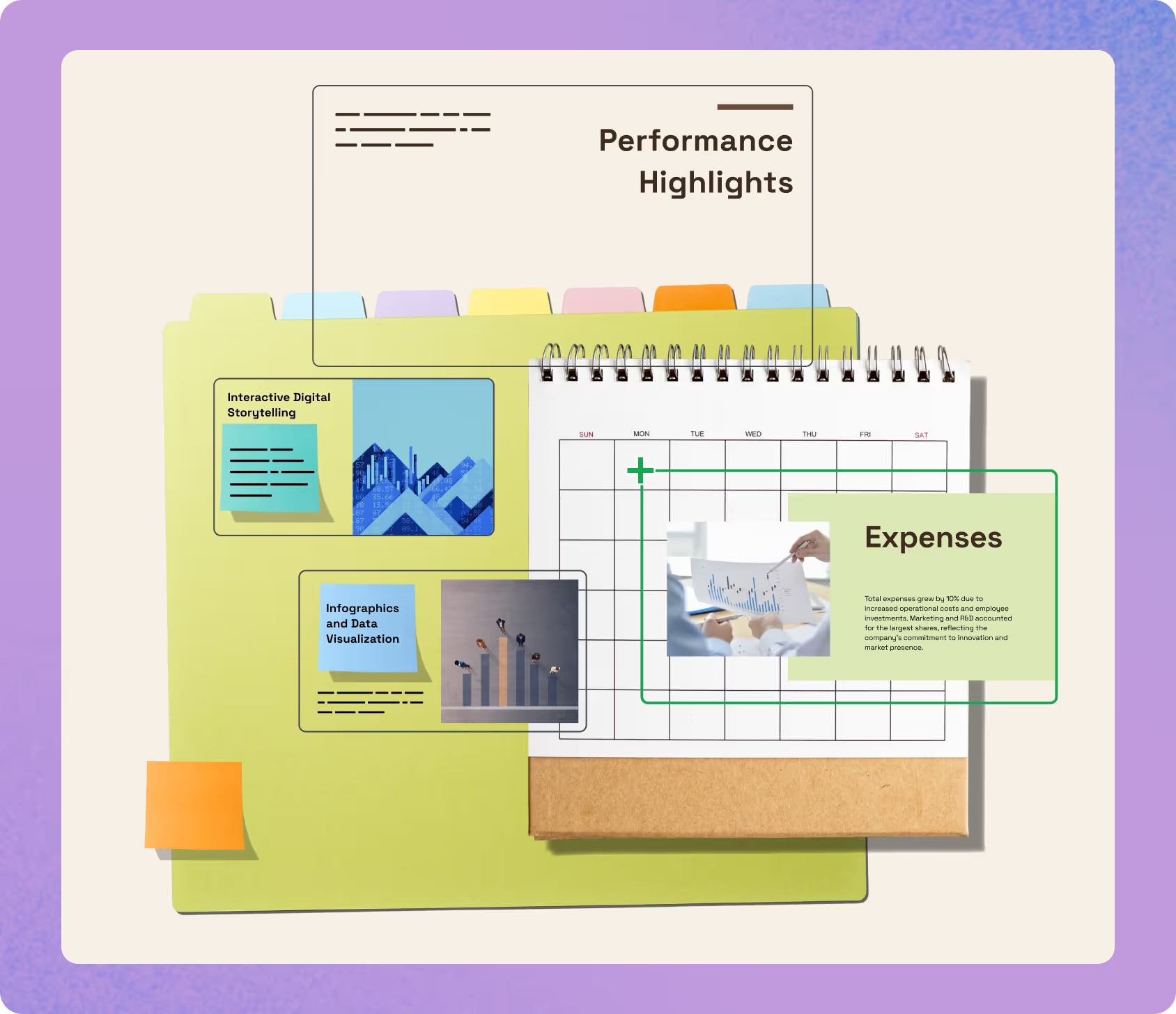
Next-gen virtual presentation tools
Take advantage of Prezi's exclusive, cutting-edge tools to elevate your video presentations. With Prezi Video, you can record your presentation, use live recording features, or apply a Prezi template to seamlessly present with video in real time.
An easy switch from slide-based tools
Prezi is fully compatible with PowerPoint files, so you don’t have to worry about losing your work from slide-based presentation tools. Just import your PowerPoint presentation or PDF into Prezi and see it in action. Adding content like text, images, animations, and videos is just as straightforward.
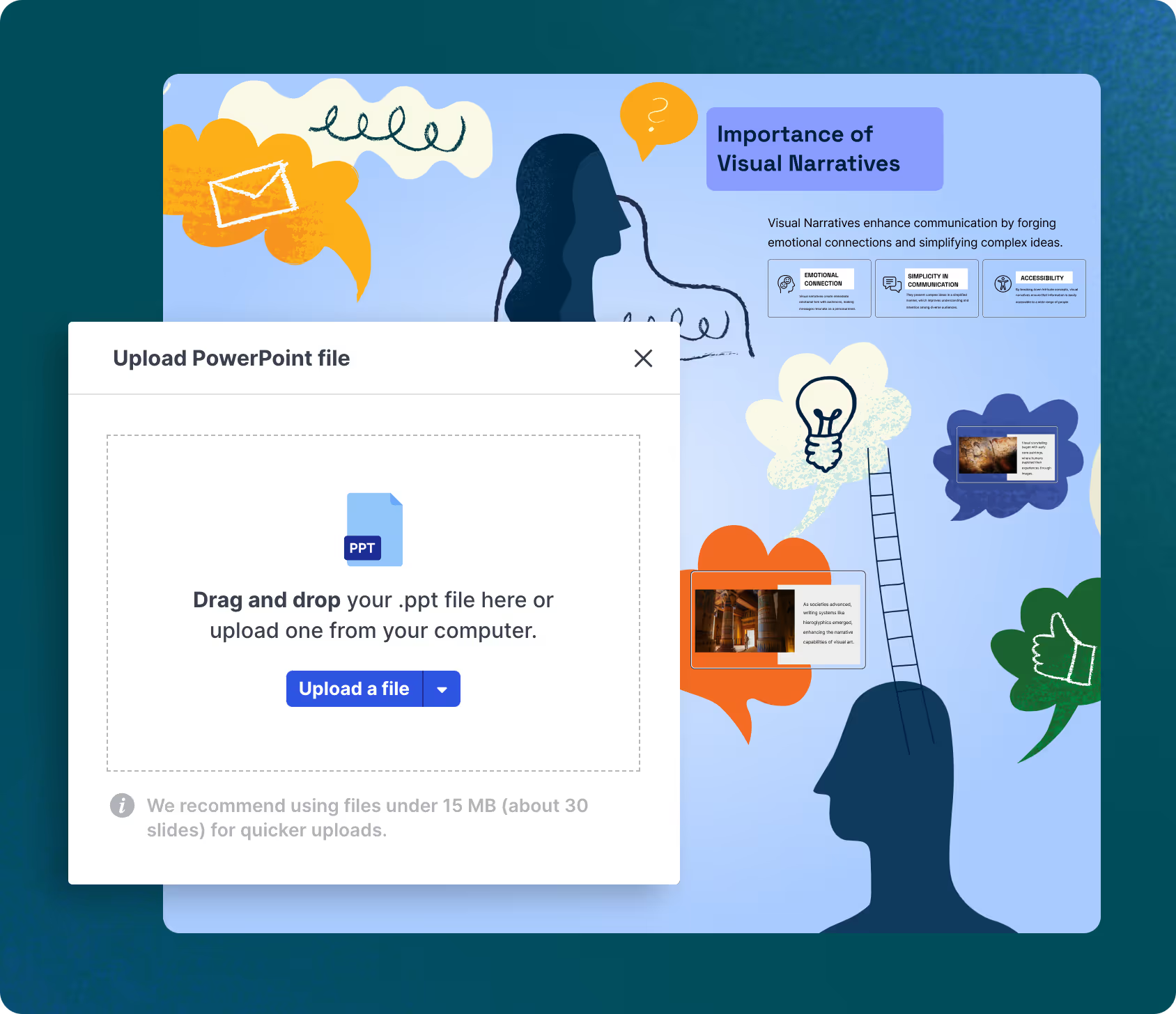
Hear from others
You don’t have to listen to us. Prezi is used by hundreds of thousands of people and companies around the world like you.
“The Prezi platform is such an engaging way to connect with students and this feature makes creating presentations so much faster!"
"Prezi AI is a game-changer….All I have to do is upload my directions, tweak a few details, and spend a little time putting my final photo touches into the presentation."
"The new Prezi AI feature gives you a great outline for new presentations based on a few words and ideas you provide. The professional look is easy to adjust to match your branding and still allows the outstanding freedom of creativity that Prezi is known for. "
Prezi AI
Make a great presentation in no time with Prezi AI
Just enter a few ideas or an outline and we’ll create your presentation for you.
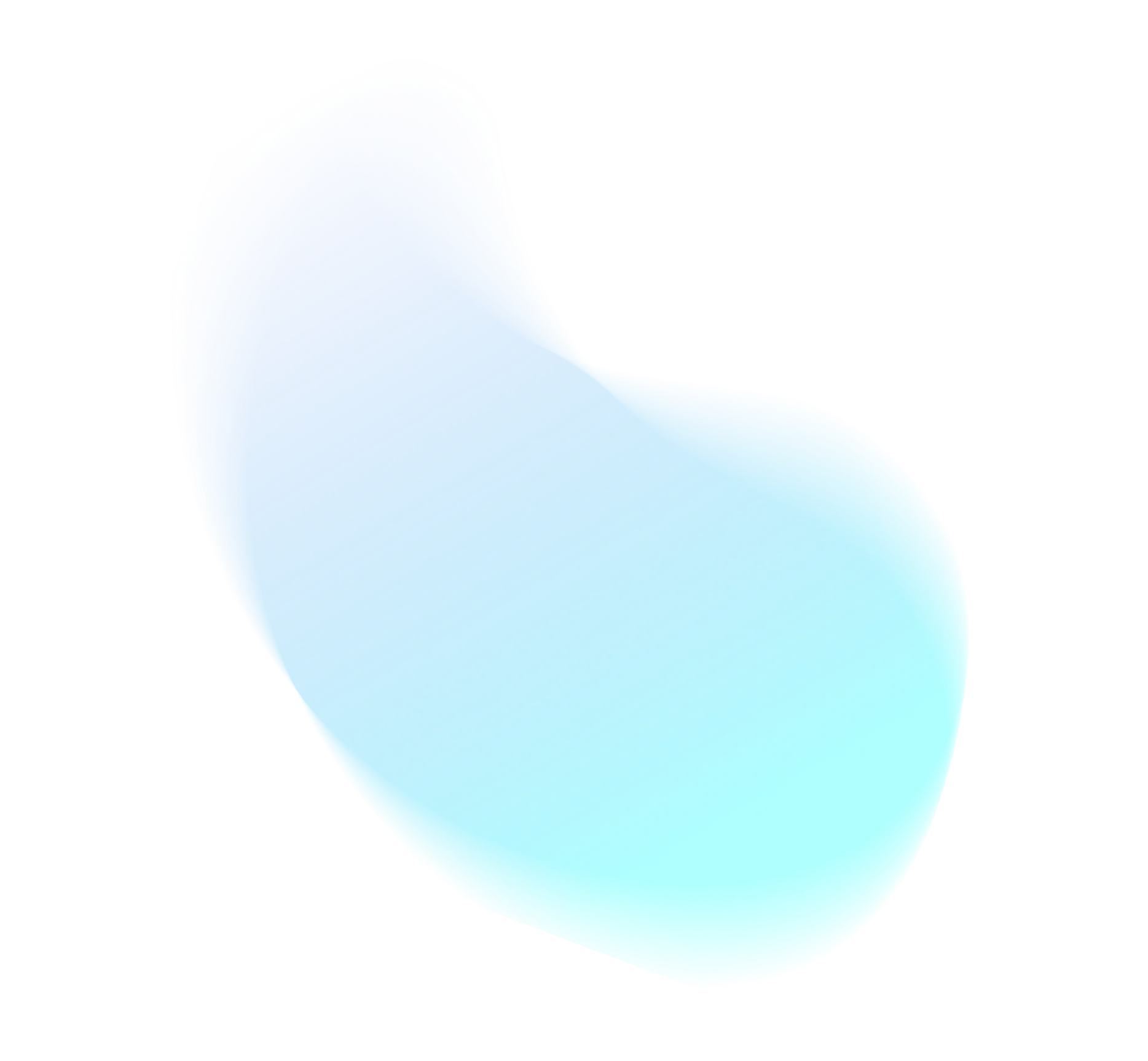
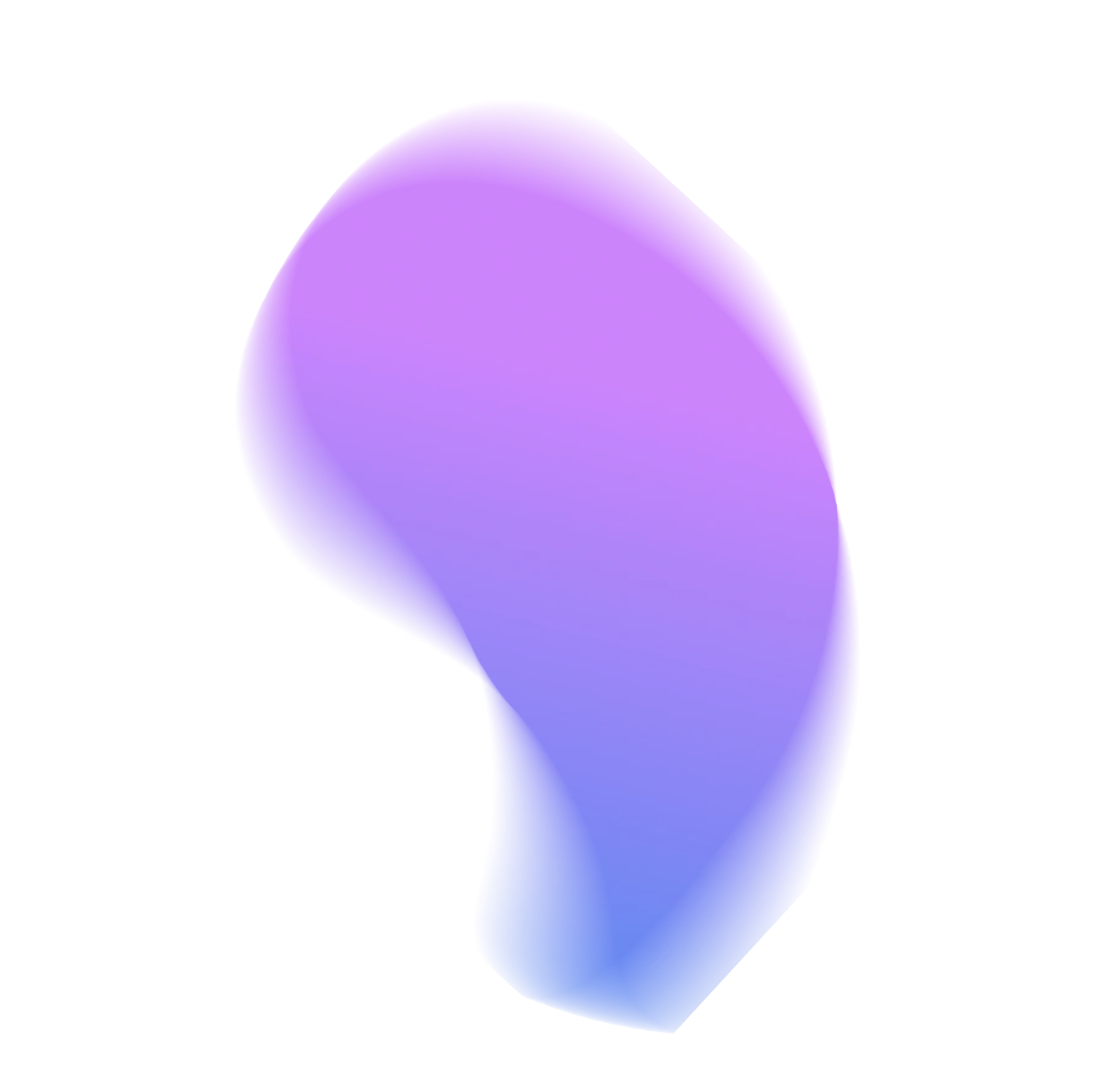
Frequently asked questions
Can I create a Prezi presentation from my PowerPoint slides?
Yes. Prezi makes it easy to transform your existing PowerPoint presentation into something dynamic. Simply upload your PowerPoint slide deck to Prezi Present, and our tools will help you convert it into an interactive Prezi presentation. You can also import files from Google Slides or Keynote by exporting them as PDFs first.
How does a Prezi presentation compare to a PowerPoint presentation?
Prezi goes beyond what a traditional PowerPoint presentation can offer. Instead of static slides, Prezi presentations are built on an open, zoomable canvas. This unique format lets you highlight your key ideas, move conversationally through your content, and create an engaging storytelling experience that captivates your audience—something a standard PowerPoint presentation just can’t do.
What makes Prezi AI better than PowerPoint AI?
Prezi AI is tailored for creating visually stunning, zoomable presentations quickly and efficiently. Unlike PowerPoint AI, Prezi AI leverages insights from the world’s largest database of successful presentations to guide you in crafting standout designs. Other AI PowerPoint generators focus on slide-based formats, but Prezi AI provides cinematic movements, zooming frames, and a map-like canvas to truly set your presentation apart.
Why is Prezi one of the best alternatives to PowerPoint?
Prezi is more than an alternative to PowerPoint—it’s a complete upgrade. With its open canvas, dynamic zooming, and intuitive storytelling features, Prezi empowers you to create presentations that are more engaging and memorable. Unlike AI PowerPoint generators, Prezi AI combines design intelligence with creative freedom to help you stand out, whether you're pitching ideas, teaching, or presenting at an event.
What is the best PowerPoint AI generator alternative?
Prezi AI is widely regarded as one of the best PowerPoint AI generator alternatives. It offers all the benefits of AI-driven content creation but goes further with features designed to inspire and engage your audience. By integrating AI tools with Prezi’s hallmark zooming and cinematic effects, Prezi AI creates presentations that far surpass what PowerPoint AI and other AI PowerPoint generators can deliver.
Can Prezi AI help me customize my presentations?
Absolutely. Prezi AI enables you to create and customize your presentation effortlessly. Upload your own images, videos, or assets, or search through millions of icons and visuals directly within Prezi. Unlike some AI PowerPoint generators, Prezi AI lets you retain full control over your style while providing intelligent recommendations to elevate your content.
Is Prezi AI included in my subscription?
Yes, Prezi AI is part of your Prezi subscription. It helps you create AI presentations with AI-powered tools for content generation, text formatting, and design. Unlike PowerPoint AI, Prezi AI also includes advanced features like cinematic zooming and storytelling elements, making it easy to craft compelling presentations.
Why choose Prezi over other AI PowerPoint generators?
Prezi isn’t just another AI PowerPoint generator—it revolutionizes the way you can create presentations. While PowerPoint AI focuses on enhancing slide decks, Prezi AI introduces a completely new way to present ideas with an open canvas, zooming transitions, and interactive design features. If you’re looking for an alternative to PowerPoint that truly stands out, Prezi is the clear choice.
How does Prezi help improve audience engagement compared to traditional slide decks?
Prezi’s open canvas and zooming capabilities are designed to captivate audiences in ways that traditional slide decks often fail to achieve. Instead of moving through a linear series of static slides, Prezi allows you to present dynamically, jumping to the most relevant points based on your audience’s interests or questions. The zooming visuals and cinematic transitions guide attention where it matters most, keeping viewers engaged and focused on your message.
Why is Prezi one of the best alternatives to PowerPoint for teams and organizations?
For teams and organizations seeking alternatives to PowerPoint, Prezi offers collaborative tools and presentation features that stand apart. Prezi’s open canvas encourages creative thinking, allowing multiple contributors to structure and visualize ideas dynamically. Prezi AI also streamlines the creation process, helping teams craft polished, engaging presentations quickly—something even the best PowerPoint AI generator struggles to match. With Prezi, your team can create presentations that are not only professional but also inspiring and memorable.
How does Prezi help me customize my presentation to match my brand or style?
Prezi gives you complete creative control over your presentation, allowing you to tailor every detail to match your brand or personal style. You can upload your own images, videos, logos, and fonts to create a fully branded experience thanks to Prezi’s Brand kit feature. We also have millions of searchable assets like icons, photos, and graphics that you can use to elevate your design. Whether you’re presenting to clients, colleagues, or a larger audience, Prezi ensures your AI presentation stands out while fully representing your unique identity.